Heart Rate Variability
Heart Rate Variability, or HRV for short, is a non-invasive measure of your autonomic nervous system, which is the body’s main control center. It is widely considered as one of the best objective metrics for physical fitness and determining your body’s readiness to perform. HRV is literally the variance in time between the beats (NN interval) of your heart.
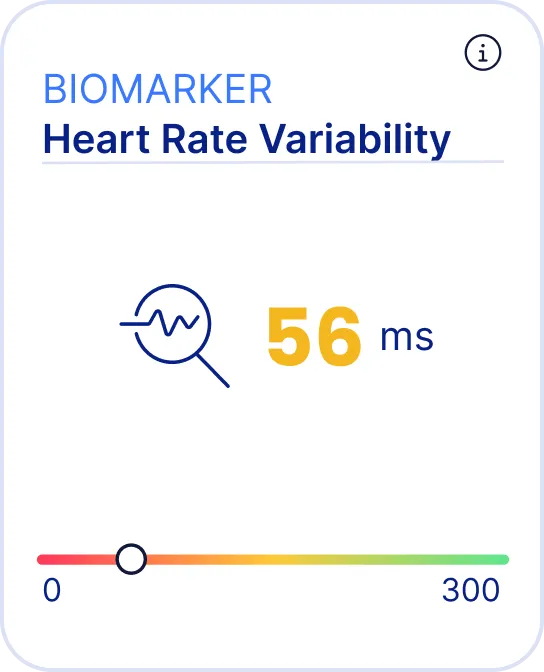
Name | Unit | programmatic name |
---|---|---|
Heart Rate Variability | Beats per minute | heart_rate_variability |
A commonly used statistical metric for representing short-time HRV (over a time duration of the order of 10s to 1 min) is the Standard Deviation of NN intervals (SDNN). It quantifies the variability between successive normal-to-normal (NN) intervals, which are the time intervals between consecutive heartbeats. In this case, PPG signals are used to extract the NN intervals. Starting from PPG signals, the following steps are executed to determine the SDNN value:
- PPG signal preprocessing
- Systolic peak detection
- NN interval calculation
- SDNN calculation
The definition and additional contextual information of each output is outlined below in the table.
The current mean absolute error of the SDNN measurement through the IntelliProve algorithms is 13 ± 15 ms (milliseconds). In 96.5% of the measurements the absolute measurement error is less than 40 milliseconds. A good correlation (r=0.89; p<0.00001) between video-based HRV measurement and ground truth HRV values (measured by using ECG signals acquired from a chest belt sensor) can be observed.
HRV is a valuable metric for assessing stress levels or recovery after sports. When a person has a low HRV, it indicates a high activity of the sympathetic nervous system (SNS), which is associated with stress, overwhelm and arousal.
Assessing the HRV at rest can provide valuable personalized insights into:
- Quantifying the chronical stress level.
- Scoring sleep quality and total wellbeing of the person. A reduction in HRV will be visible when sleep quality is reduced.
- The impact of unhealthy food or low nutritions.
- The quality and effects of recovery exercises. Engaging in activities that aim to increase HRV and restore the balance of the autonomic nervous system (ANS) can be beneficial for recovery. The metric should be carefully measured and controlled to ensure the positive effect of the training and the needed personalized durations.
Use HRV as a powerful metric to assess body stress load during a health check-in. Provide personalized recommendations based on the corresponding score level.
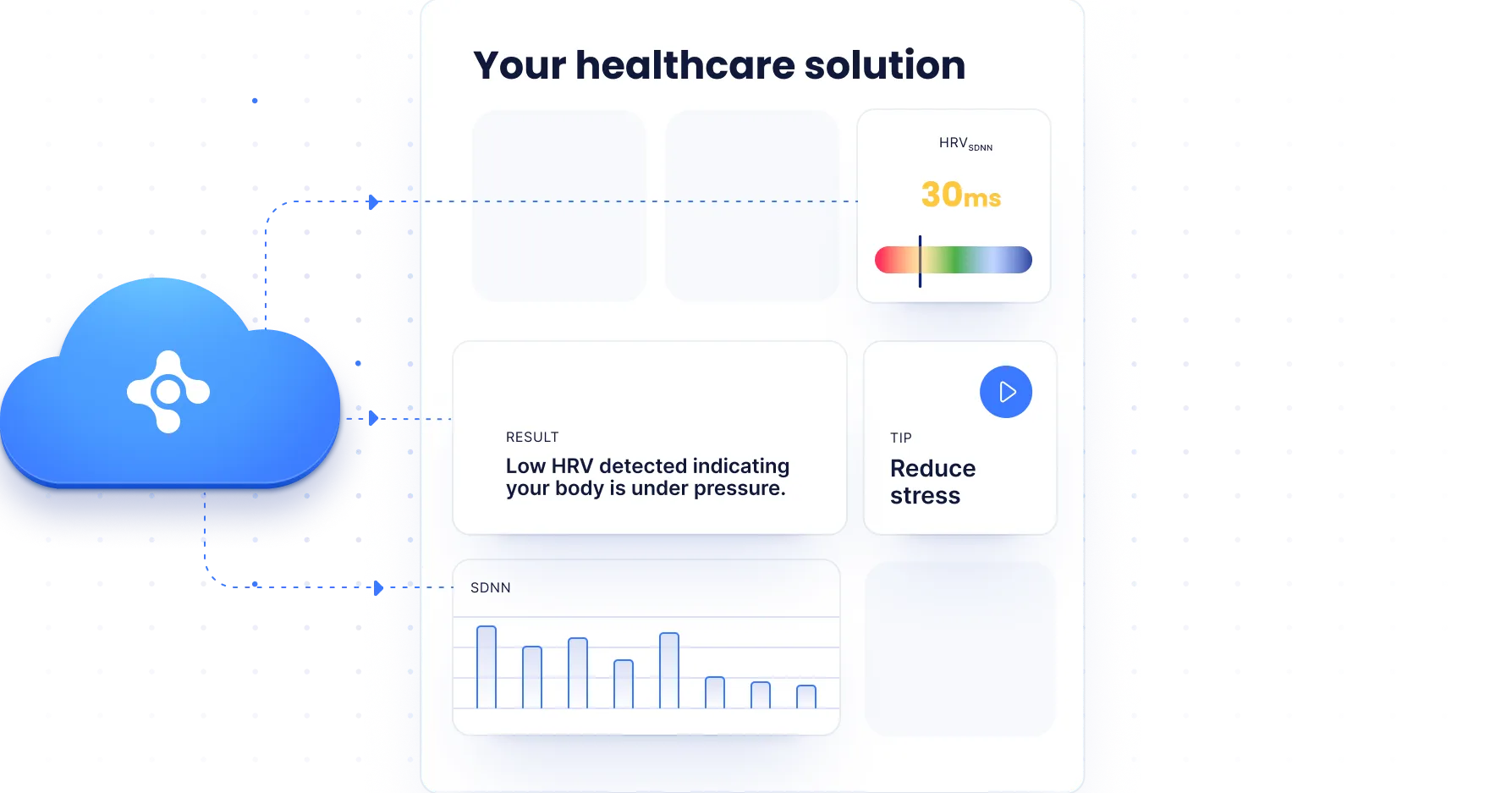
Output (milliseconds) | Definition | Example Color Indicator | Example User Text |
---|---|---|---|
0 - 35 | Below average | Red | Your heart rate variability value is below average. This indicates your body is under pressure for some reason which could be due to exercise, psychological proceedings or any other external and internal stressors. |
36 - 100 | Average - Healthy | Yellow | Your heart rate variability is in a normal range. To further increase your heart rate variability you can perform aerobic and/or breathing exercises or meditation. |
>100 | Above average – Excellent | Green | Your heart rate variability is above average which general states that the body has a stronger capability to cope up with stress or is recovering strongly from previously built-up stress. |
- Pinheiro N, Couceiro R, Henriques J, Muehlsteff J, Quintal I, Goncalves L, Carvalho P. Can PPG be used for HRV analysis? Annu Int Conf IEEE Eng Med Biol Soc. 2016 Aug;2016:2945-2949.
- Odinaev I, Wong KL, Chin JW, Goyal R, Chan TT, So RHY. Robust Heart Rate Variability Measurement from Facial Videos. Bioengineering (Basel). 2023 Jul 18;10(7):851.
- Yu SG, Kim SE, Kim NH, Suh KH, Lee EC. Pulse Rate Variability Analysis Using Remote Photoplethysmography Signals. Sensors (Basel). 2021 Sep 17;21(18):6241.
- Martinez-Delgado GH, Correa-Balan AJ, May-Chan JA, Parra-Elizondo CE, Guzman-Rangel LA, Martinez-Torteya A. Measuring Heart Rate Variability Using Facial Video. Sensors (Basel). 2022 Jun 21;22(13):4690.