Mental Health Risk
A unique holistic approach to assess the risk of an individual to develop a mental health condition or issue over time. A mental health condition is characterized by a relevant disturbance in an individual’s cognition, emotional regulation, or behaviour.
The mental health risk is a clinically-validated biomarker benchmarked to questionnaires to assess the mental health status of the individual in terms of physiological stress and anxiety (PHQ-9, GAD-7, CSAI-2).
The IntelliProve processing engine uses a combination of machine learning informed by selected HRV metrics, facial micro expression patterns and context-based reasoning over time to determine the Mental Health Risk. This metric lets the user know if their risk of mental health conditions is low, medium, or high compared to their baseline.
When working preventively, it is crucial to identify potential negative trends. While experiencing one bad day may not indicate a high risk of developing a mental health condition, an ongoing series of negative experiences or a long-term downward trend can be critical. A common example of this is individuals who are on the path to burnout. They may start accumulating stress, leading to a decline in sleep quality, and often remain in denial about their feelings until a breaking point occurs. With the Mental Health Risk initiative, our aim is to raise awareness among users about the possibility of a breakdown and encourage early intervention.
Providing users with a risk score will raise awareness about potential concerns, such as moving towards a long-term absence. When a high risk is detected, users can be recommended to schedule a call with a therapist for further support and guidance.
This risk assessement is the ideal (more objective) biomarker in addition to subjective user reporting.
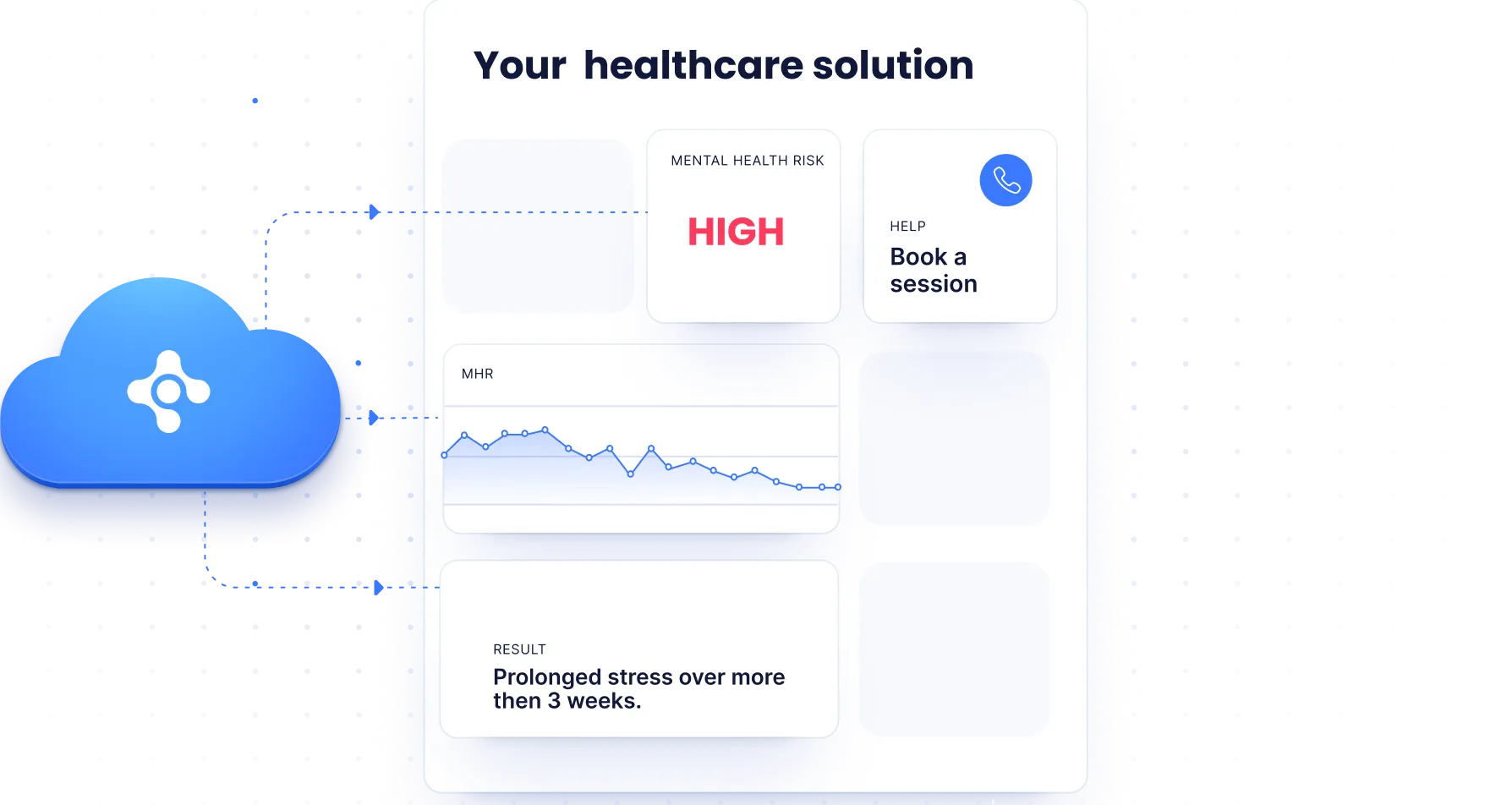
Mental Health Risk is returned as a part of the output response JSON, with the key ‘mental_health_risk’.
Output | Definition | Example Color Indicator | Example User Text |
Null | Insufficient data | Grey | Not enough data present to calculate Mental Health Risk. This value is based on history of up to 3 weeks in the past. |
1 | Low mental health risk | Green | You have a lower risk of mental health conditions. |
2 | Medium mental health risk | Yellow | You have a medium risk of mental health conditions. |
3 | High mental health risk | Red | You have a higher risk of mental health conditions. We recommend that you pursue a further discussion of your situation with a physician or mental health provider. |